What machine learning and rich historical data mean for fraud protection in retail
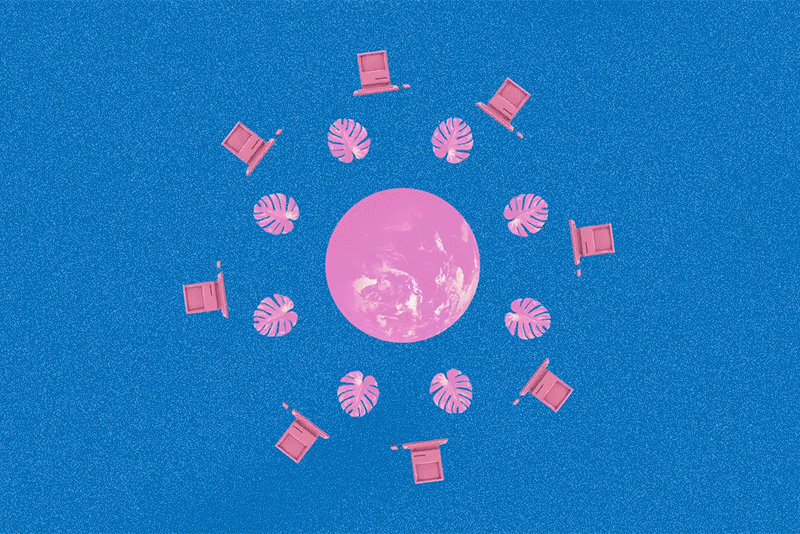
Simon Banks, Director of Merchant Services, PayPal Australia
Fraud is evolving, and many Australian businesses may struggle to keep up with fraudsters who are continuing to find new ways to evade detection and exploit vulnerabilities. In the twelve months to June 2021 alone, the Australian Payments Network[CJ1] found fraud on payment card transactions totalled $490.1 million, an increase of 9.2 per cent from the year before. Further, research from Statista s[CJ2] hows that as of 2021, around 1.25 million dollars had been lost in online shopping scams in Australia.
For retailers of all sizes, it has never been more important to get ahead and proactively find a solution that helps to stop fraudulent transactions without turning away legitimate customers and limiting opportunities for growth.
What your business needs, however, depends on the size of your organisation or the trajectory of growth that you are on. Therefore, it can be effective to look for fraud protection partners who offer tiered solutions that tailor to your business needs.
Smaller retailers and SMBs (Small to Medium sized Business), do not have the resources to hire substantial risk teams to build sophisticated risk strategies and customise their own rules. In these cases, fraud protection tools that have a fixed set of rules, can be setup easily and are affordable, are often preferred. Conversely, companies with more established risk teams often require custom tools that can match the intricacies of their organisation.
It’s important then, that businesses partner with a fraud prevention vendor that fits their current requirements and can scale to their needs.
Balancing fraud prevention with revenue
Consumers, now more than ever, demand a seamless and safe online experience and may abandon shopping carts when faced with too much friction, including friction intended to stop fraudsters.
Satisfied customers are more likely to become return customers, and it’s important to quickly differentiate legitimate customers from bad actors. Technologies are available that enable you to make that distinction automatically and invisibly, and drive customer satisfaction by ensuring ‘false positives’ don’t stop the vast majority of legitimate customers from purchasing.
False positives occur when a fraud engine thinks a legitimate transaction looks ‘wrong’ and rejects it and can lead to good customer orders being lost to competitors. For example, a legitimate customer, with a legitimate credit card, might place an order from a device they don’t usually use, or from a location/IP address they haven’t used before. This could trigger less sophisticated fraud systems and a legitimate order could be rejected
Accuracy is key. Technologies today can quickly give analysts the capabilities they need to make more accurate decisions on transactions. At PayPal, our graph-based case management dashboard, which allows retailers to visually depict how transactions are linked through shared attributes. This means retailers can better analyse and understand transactions as well as automatically approve, decline or flag transactions based on unique filters. These dashboards also allow for the creation of specific custom fields, such as a store number or SKU, which can be applied to filters.
Rich data and machine learning
Getting risk prevention right means reducing chargebacks, lessening friction at checkout, and increasing conversion with customer satisfaction. Outside of transactions, it also improves brand reputation and reduces manual processes.
The biggest value-add when it comes to tapping into the right technology to support your risk management strategy is the substantial amount of historical transaction data from which machine learning and artificial intelligence can develop unique risk rules and algorithms. Device fingerprinting, for example, is a powerful machine learning-driven technology, which is stateless and remains stable regardless of changes made by fraudsters.
Using machine learning, fingerprinting can detect fraud before it happens and reveal risk before the bad actor does — so merchants can decide whether to accept or reject a transaction within seconds. It does not require personal data, as device ID uses anonymous computer characteristics and returning devices are verified instantly in real time by their unique characteristics. If there is any correlation or pattern between the device and fraudulent behaviour, it will result in a high-risk score and the transaction will be rejected.
Paving the way for success
Digital fraud is evolving, and fraudsters are getting more sophisticated. Getting the right balance between risk and revenue by leveraging technology is critical and can mean the prevention of lost sales, increased business growth, and a protected brand reputation.
There are tiered fraud protection offerings out there that provide solutions for diverse needs under one roof. Look for the solution that works for you both now and that will scale up as needed in the future. Technologies that leverage large historical data sets to inform machine learning and artificial intelligence will naturally make more informed and accurate fraud decisions. There are lots of out of box solutions out there with machine learning built in – but it is the richness of the data that can make the most difference.
At the forefront of digital commerce, PayPal manages the safety and fraud prevention for the daily online commerce needs of more than 430 million active online users. Our dedicated team of anti-fraud specialists provide progressive system upgrades, real-time transaction monitoring and extensive data encryption to keep you ahead of fraudsters.
[CJ1]Publicly available data
[CJ2]Publicly available data